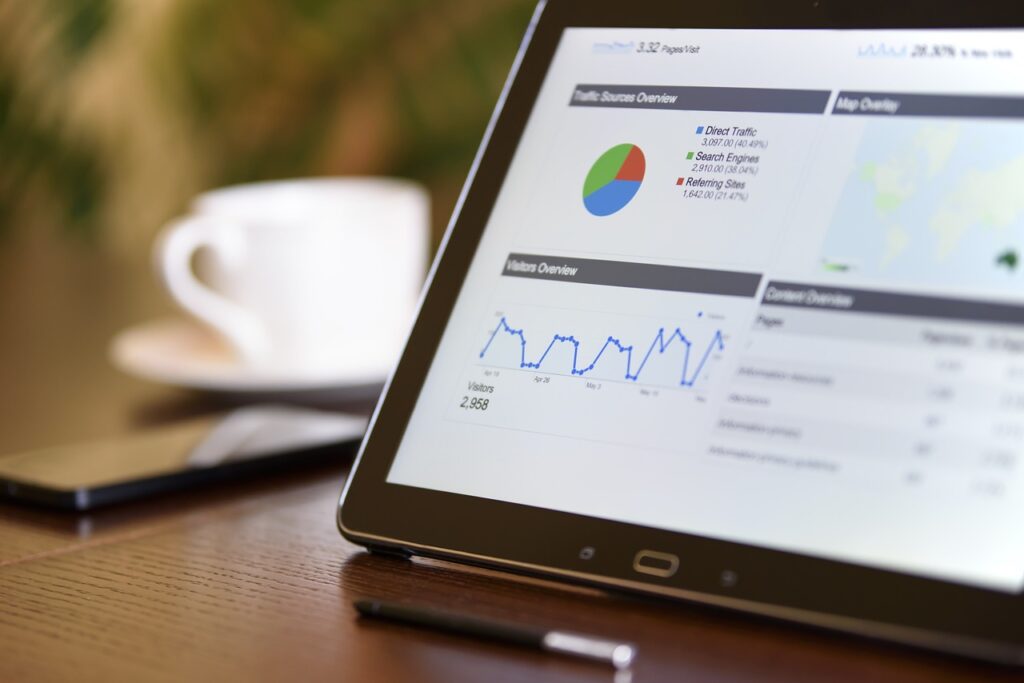
While 68% of marketers are already using AI in their daily work, the reality of AI in marketing isn’t as rosy as vendors would have us believe. Despite the growing adoption, only 4% of marketers believe AI will create more jobs in our field, and 40% actually expect a decline in work quality and creativity.
These statistics tell us an important truth: the impact of AI on marketing isn’t playing out as many predicted. In fact, we’re seeing a significant gap between AI’s promised potential and its real-world results. As marketing leaders ourselves, we’ve witnessed countless brands rush into AI implementation without understanding the hidden pitfalls that can derail their efforts.
In this article, we’ll expose the uncomfortable truths about AI marketing tools and examine why many brands’ AI initiatives fail to deliver expected results. We’ll also explore what you need to know to avoid these common pitfalls and make informed decisions about your marketing technology investments.
The False Promise of AI Marketing Automation
Marketing technology vendors paint a rosy picture of plug-and-play AI solutions, but the reality often falls short of expectations. Many businesses discover that implementing AI marketing tools requires more than just purchasing software and pressing “start.”
Why plug-and-play AI solutions often disappoint
Plug-and-play AI solutions promise quick implementation and immediate results, but they frequently disappoint due to fundamental limitations. These tools typically work with disconnected marketing systems, leading to significant data loss and delayed responses. Furthermore, businesses often face integration challenges with existing platforms, making it difficult to achieve the promised efficiency gains.
Hidden costs and resource requirements
The financial impact of AI marketing implementation extends far beyond the initial purchase. Here are the often-overlooked expenses:
- Setup and onboarding fees for system configuration
- Ongoing training and support services
- Custom feature development costs
- Integration expenses with existing CRM systems
Notably, while smaller businesses might find integrated solutions cost-effective initially, larger organizations frequently encounter higher implementation costs due to complex integration requirements. Subsequently, many companies discover they need specialized personnel to manage these systems effectively.
The myth of fully automated marketing
One of the most persistent misconceptions is that AI marketing automation runs entirely on its own. Consequently, marketing teams often underestimate the human involvement required for success. The truth is that marketing automation demands a well-thought-out strategy and continuous human oversight.
Marketing automation isn’t a system for passive marketers – it’s a tool that requires smart, active management. Primarily, your role as a marketer involves analyzing and evaluating results to fine-tune content and strategy. The system helps process vast amounts of data, but it needs human guidance to deliver engaging, personalized content based on customer interests.
Data quality remains a critical factor, as AI systems can only be as good as the information they’re trained on. Poor quality data inevitably leads to unreliable results and low-impact campaigns. Additionally, 69% of consumers support using AI to improve customer experience, making it crucial to maintain transparency about how data is collected and used.
Data Quality and Integration Nightmares
Quality data forms the backbone of successful AI marketing implementations, yet many organizations struggle with this fundamental aspect. Almost 40% of projects fail specifically because of difficulties in integrating different data sets.
Poor data leading to poor AI decisions
Poor-quality data significantly impairs AI’s functionality, leading to a cascade of negative outcomes. When marketing teams feed AI systems with inaccurate or incomplete information, the consequences manifest in several ways:
- Inaccurate targeting affecting 30% of campaigns
- Customer loss impacting 29% of businesses
- Lead generation failures occurring in 28% of cases
- Reduced productivity affecting 27% of operations
- Wasted marketing spend in 28% of instances
Integration challenges with legacy systems
Legacy systems present particularly complex obstacles for AI marketing implementation. Primarily, these systems operate in data silos, making valuable information inaccessible across departments. Moreover, most legacy platforms run on outdated programming languages and databases that clash with AI’s need for advanced data processing and real-time analytics.
Specifically, the hardware supporting legacy systems often lacks the computational power required for AI operations. Integration efforts frequently demand extensive customization, sometimes requiring complete overhauls of existing IT infrastructure.
Data privacy and compliance pitfalls
Privacy concerns have emerged as a critical challenge, with 82% of consumers expressing worry about how AI uses their personal information in marketing and customer service. Organizations must navigate strict regulatory frameworks, particularly GDPR, which has fundamentally changed how companies collect and store customer data.
The stakes are particularly high when dealing with sensitive information. Unauthorized access or exploitation of shared data can result in severe consequences. Furthermore, AI systems that collect data from multiple sources may inadvertently create personal identifiable information (PII), requiring additional protection measures.
Ultimately, the combination of poor data quality, integration challenges, and privacy concerns creates a complex web of issues that many organizations underestimate. Without addressing these fundamental problems, AI marketing initiatives risk not only failing but potentially damaging customer trust and brand reputation.
The Human Factor in AI Marketing
Behind every successful AI marketing implementation stands a crucial element – the human workforce. Recent studies reveal a striking gap between executive enthusiasm and ground-level acceptance of AI marketing tools. Only 33% of entry-level marketers feel confident in AI’s ability to improve marketing, compared to 55% of marketing executives.
Resistance to change from marketing teams
Sales and marketing teams often show reluctance toward AI-driven processes, primarily because they view these tools as threats rather than enablers. This resistance manifests through slow adoption rates of AI-powered tools, although these solutions could enhance targeting and campaign performance. According to industry research, although marketing executives want minimal human oversight, only 5% of entry-level marketers support a fully automated approach.
Lack of AI literacy among marketers
The knowledge gap in AI marketing continues to widen, creating significant barriers to adoption. Here’s what recent data tells us:
- 67% of respondents cite lack of education as the primary barrier to AI adoption
- 75% of organizations either have no AI-focused education (47%), are developing it (24%), or remain uncertain about its existence (4%)
- 66% of companies wouldn’t hire someone without AI literacy skills
This literacy gap puts businesses at a competitive disadvantage, hindering their ability to harness AI’s potential for data-driven decision-making and targeted campaigns.
Over-reliance on AI without human oversight
Although AI excels at analyzing trends, human intuition remains irreplaceable for understanding consumer behavior nuances. Marketing teams that depend too heavily on AI-generated insights risk losing the creative spark that ignites compelling campaigns. Human oversight ensures that AI aligns with ethical guidelines and prevents potential issues like:
- Inadvertent creation of offensive advertisements
- Propagation of biases in marketing content
- Loss of authentic customer connections
Human marketers understand their audience on a deeper level, producing emotionally appealing content that resonates authentically. Through regular audits and reviews, human oversight helps maintain quality, originality, and relevance in AI-generated marketing materials.
Implementation and Strategy Misalignment
Success with AI marketing tools demands careful planning, yet many organizations rush into implementation without establishing clear objectives. A recent study reveals that one-fifth of CMOs prioritize AI development as their primary focus for the next year, primarily driven by competitive pressure rather than strategic necessity.
Rushing AI adoption without clear goals
The excitement surrounding AI often leads to hasty implementations without a clear path to return on investment. Organizations frequently discover that AI solutions require substantial effort and time to yield tangible business improvements. Hence, deploying technology before it reaches sufficient maturity creates unnecessary complications and risks.
Failure to align AI with marketing strategy
AI itself should never be the end goal – instead, it must serve as a tool to achieve broader business objectives. Nevertheless, many companies implement AI solutions without analyzing their current marketing workflows or identifying specific areas where AI can add value.
To maximize AI’s potential, organizations should:
- Define marketing objectives that AI will support
- Analyze existing workflows for AI integration opportunities
- Select solutions aligned with specific business needs
- Establish robust data privacy guidelines
Missing KPIs for AI success measurement
Ultimately, the absence of proper measurement frameworks undermines AI marketing initiatives. Studies indicate that model-based metrics remain experimental, with many organizations still relying on human evaluators for manual assessment. Furthermore, improving one KPI can sometimes negatively impact another, making comprehensive measurement crucial.
Operational metrics play a vital role in connecting technical model quality with financial outcomes. These measurements help organizations understand whether their AI initiatives generate tangible value. For instance, tracking cost savings, employee productivity, and compliance rates provides concrete evidence of AI’s impact on business operations.
The growing need to quantify AI’s impact and demonstrate ROI has become increasingly important. Organizations must establish specific metrics that measure both direct and indirect benefits of their AI implementations. This includes monitoring scalability, market share growth, and customer retention rates to assess the overall effectiveness of AI marketing initiatives.
Common AI Marketing Tool Selection Mistakes
Selecting the right AI marketing tools requires careful consideration and thorough evaluation. Recent data shows that 97% of business leaders believe AI will enable better analysis of marketing data, yet many organizations struggle with tool selection and implementation.
Choosing tools without proper evaluation
Proper evaluation of AI marketing tools demands more than just feature comparison. Research indicates that businesses often overlook critical assessment criteria when selecting AI solutions. Here are the essential factors to consider:
- Technical requirements and infrastructure compatibility
- Integration capabilities with existing marketing stack
- Data security and compliance standards
- Total cost of ownership, including maintenance
- Vendor reputation and market adoption rates
- Customer support quality and availability
Overlooking scalability requirements
Fundamentally, scalability ensures that AI systems can grow alongside your business needs. Many organizations presently face performance degradation and increased costs when their AI solutions fail to scale effectively. Scalability challenges primarily manifest in three areas:
First, data management becomes increasingly complex as businesses expand. Without proper infrastructure, organizations struggle to handle larger datasets efficiently. Second, performance issues emerge when systems cannot adapt to growing workloads. Third, costs often spiral out of control when scaling requirements weren’t considered during initial selection.
Ignoring vendor lock-in risks
Vendor lock-in poses a significant threat to long-term marketing success. Essentially, more than 80% of organizations face vendor lock-in issues, making it crucial to understand these risks before committing to any AI marketing solution.
Lock-in challenges markedly affect businesses through:
Limited flexibility and innovation: Being tied to a single vendor restricts access to new technologies and innovations. Organizations find themselves confined to the solutions and updates offered by their locked-in vendor, potentially missing opportunities for advancement.
Escalating costs: Once dependent on a vendor’s products, businesses often face price increases without viable alternatives. This situation leaves organizations with minimal negotiation power and potential budget overruns.
Data accessibility issues: Many vendors use proprietary data formats, making it difficult to extract information for use in other systems. This limitation can create significant obstacles when attempting to switch providers or integrate with new tools.
To minimize these risks, organizations should:
- Prioritize solutions that adhere to widely accepted standards and protocols
- Embrace open standards platforms where possible
- Negotiate flexible terms of service with clear exit strategies
- Maintain relationships with multiple vendors
Ultimately, successful AI marketing tool selection requires a balanced approach that considers immediate needs while planning for future growth. Organizations must simultaneously evaluate technical capabilities, scalability potential, and long-term implications of vendor relationships to make informed decisions that support their marketing objectives.
Conclusion
Success with AI marketing tools demands more than blind adoption of trending technology. Through our analysis, we’ve seen how rushed implementations, poor data quality, and misaligned strategies lead many organizations to disappointing results.
Most compelling evidence shows that effective AI marketing requires a balanced approach. Companies must address fundamental challenges like data integration, team resistance, and proper measurement frameworks before expecting meaningful returns from their AI investments.
Rather than viewing AI as a replacement for human marketers, smart organizations treat it as a powerful complement to human creativity and insight. This mindset shift, combined with careful tool selection and strategic alignment, makes the difference between AI marketing success and failure.
Businesses that thrive with AI marketing focus on three key elements: quality data management, comprehensive team training, and clear performance metrics. These organizations understand that AI serves as a tool to enhance their existing marketing capabilities, not a magical solution that runs itself.
Looking ahead, AI marketing will certainly evolve, but its success will always depend on thoughtful implementation and human oversight. Companies that acknowledge these realities and plan accordingly stand the best chance of realizing AI’s true potential in their marketing efforts.