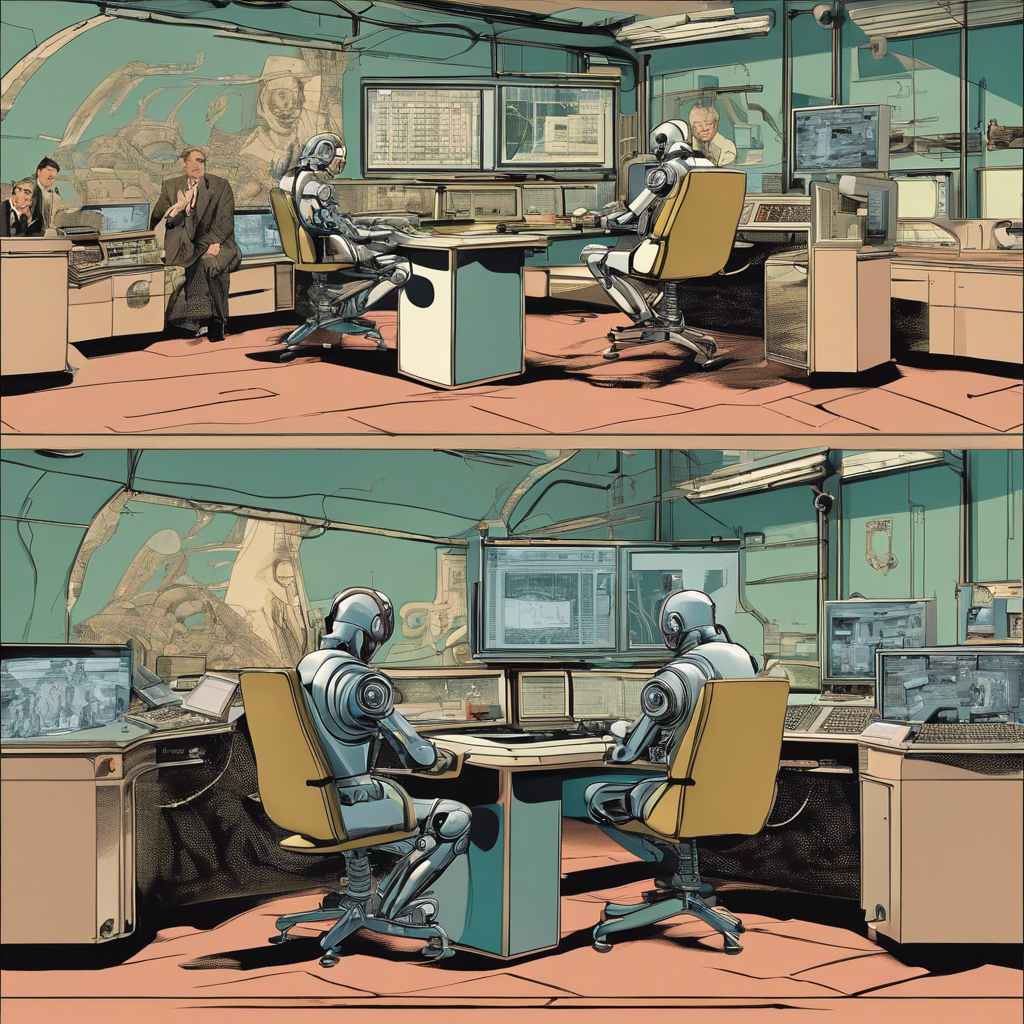
Experts predicted we would have self-aware robots and superintelligent machines by 2020, yet the future of artificial intelligence and AI futures have taken a dramatically different path than anyone anticipated. While AI has transformed our world, it hasn’t done so in the ways most specialists forecasted.
The development of AI has surprised experts across multiple domains. For instance, AI futures trading evolved far more rapidly than expected, with sophisticated trading bots and software becoming mainstream years before predictions suggested. However, other areas like general AI and cognitive computing have progressed much slower than forecasted.
This article examines the most significant AI predictions that experts got wrong, exploring why these misconceptions occurred and what they tell us about technology’s actual development path. We’ll analyze everything from early timeline estimates to unexpected breakthroughs that nobody saw coming.
The Early Years: Overestimating AI’s Timeline
The summer of 1956 marked a pivotal moment in artificial intelligence history when a group of researchers gathered at Dartmouth College, officially coining the term “artificial intelligence”. Their optimism about AI’s potential sparked a wave of ambitious predictions that would shape the field for decades.
1950s-1970s: The Age of Optimism
The pioneers of AI made bold claims about machine capabilities. Herbert Simon and Allen Newell predicted in 1958 that within a decade, a computer would become the world’s chess champion and prove important mathematical theorems. Additionally, Marvin Minsky declared in 1967 that the challenge of creating artificial intelligence would be solved within a generation.
Why Early Pioneers Got the Timeline Wrong
The early enthusiasm stemmed from initial successes with basic computing tasks. Nonetheless, researchers had grossly underestimated the complexity of human intelligence. The first-generation AI systems, though innovative, faced severe limitations in computing power. Furthermore, training the first artificial neural network, Perceptron Mark I in 1957, required around 700,000 operations to perform simple visual tasks.
Lessons from Early AI Winters
The consequences of overoptimism became apparent in 1973 when the Lighthill Report critically evaluated AI research. The report concluded that AI had failed to achieve its ambitious objectives, leading to significant funding cuts from the British government. Consequently, DARPA, which had been generously funding AI research, also reduced its support.
The first AI winter, spanning from 1974 to 1980, emerged as a direct result of unfulfilled promises. This period taught valuable lessons about the gap between theoretical possibilities and practical achievements in artificial intelligence development. The setback wasn’t merely financial – it created a lasting impact on how researchers approached AI development, introducing a more measured and realistic perspective to the field.
Failed Predictions About AI Capabilities
Voice recognition systems and self-driving cars stand as prime examples of AI capabilities that fell short of 2020 predictions. Voice-based AI solutions still struggle outside controlled environments, primarily facing challenges in reliability and real-world applications.
The Myth of General AI by 2020
Despite ambitious forecasts, general AI remains notably distant from reality. Rather than achieving human-like intelligence, AI has evolved into specialized prediction machines. In fact, today’s AI systems excel at specific tasks but lack the broader reasoning and judgment capabilities many experts anticipated by 2020.
Why Expert Timelines Keep Shifting
The uncertainty in AI development timelines stems from fundamental disagreements among experts. Some researchers anticipate breakthrough developments within months, although others project decades of work ahead. This wide disparity reflects the field’s inherent unpredictability, with most recent median predictions falling between 10-40 years for transformative AI.
Understanding the Complexity Challenge
The gap between predictions and reality emerges from several critical factors:
- Data quality and quantity significantly impact AI performance
- AI systems often fail when tasked beyond their training data
- Current models lack robust human reasoning abilities
- AI tends to generate plausible-sounding but factually incorrect content
Instead of replacing human intelligence, AI has emerged as an amplification tool for human capabilities. The successful implementation of AI systems depends heavily on human judgment, particularly in determining appropriate applications and assessing potential risks. Despite advances in machine learning and cloud computing, the productivity impact of emerging technology remains less dramatic than initially projected.
Unexpected Developments in AI Futures Trading
The financial sector witnessed an unexpected surge in AI trading systems adoption, with over 70% of financial institutions heavily investing in autonomous risk management methods by 2020 [2]. This development marked a significant departure from traditional algorithmic trading approaches.
The Surprise Success of AI Trading Systems
Modern AI trading platforms excel at processing both structured and unstructured data simultaneously, analyzing over a million data points daily. Moreover, these systems demonstrate remarkable capabilities in sentiment analysis, scanning news articles, social media discussions, and blockchain data to adjust trading strategies accordingly.
How Trading Bots Evolved Differently Than Predicted
In contrast to earlier expectations of simple automation, trading bots developed sophisticated capabilities including:
- Real-time market sentiment analysis through natural language processing
- Dynamic risk assessment and portfolio adjustment
- Automated pattern recognition across multiple timeframes
- Integration with blockchain technologies for enhanced security
Subsequently, these advancements led to improved market efficiency and more accurate price discovery. The International Monetary Fund acknowledges that AI has been impacting financial markets for many years, primarily through sophisticated analytical methods that enhance returns for investors.
Current State of AI Futures Trading Software
The present landscape of AI futures trading software demonstrates remarkable sophistication. Advanced systems now employ quantum machine learning for complex probability calculations. In addition to this, modern platforms integrate features like statistical arbitrage and spread normalization to optimize trades.
The evolution continues as trading platforms increasingly utilize large language models to analyze earnings call transcripts and social media posts in real-time. Accordingly, major financial institutions have recognized the potential, with companies like JPMorgan and Goldman Sachs employing machine learning techniques to refine risk assessment models and predict potential faults in trading strategies.
The Social Impact Miscalculation
Recent research reveals a striking contrast between predicted and actual AI impacts on employment. Studies show that companies have been surprisingly slow to adopt AI technologies, with actual transformation occurring at a much slower pace than anticipated.
Job Displacement Predictions vs Reality
The reality of AI’s impact on employment differs markedly from early forecasts. Presently, unemployment rates for AI-exposed jobs generally track with the broader labor market. PwC projected that 7 million existing jobs would be replaced by AI from 2017-2037, yet essentially predicted 7.2 million new positions would be created.
Unexpected Ways AI Integration Occurred
The pattern of AI adoption has shown remarkable variation across industries. Primarily, younger and less-experienced workers embrace AI tools more readily, with usage declining by 0.7 percentage points for each year of occupational experience. The video game industry, undoubtedly, leads in AI adoption compared to TV, film, or music sectors.
The Human Factor Experts Overlooked
A crucial oversight in early predictions was the human element in AI implementation. Overall, AI excels at automating routine tasks but struggles with activities requiring:
- Emotional intelligence and complex decision-making
- Critical thinking and creative problem-solving
- Adaptation to unpredictable situations
Whether in healthcare, customer service, or education, human interaction remains irreplaceable. Research indicates that AI generally augments human capabilities rather than replacing them entirely. Soon, the focus will shift toward developing skills that complement AI technologies, as complete worker replacement appears unlikely in the foreseeable future.
Conclusion
Predictions about artificial intelligence have consistently missed the mark over the past several decades. Rather than achieving human-like consciousness or causing massive job displacement, AI has carved its own unique path. Trading systems exemplify this reality, surpassing expectations while general AI capabilities remain far behind initial forecasts.
Experience teaches us that AI development follows neither the optimistic timelines of early pioneers nor the pessimistic warnings of critics. Though AI has not replaced human workers en masse, it has become an essential tool across industries, particularly in specialized applications like financial trading and data analysis.
Most significantly, artificial intelligence has emerged as a complement to human capabilities instead of a replacement. This partnership between human insight and machine processing power points toward a future different from both early utopian and dystopian predictions. Success with AI requires understanding its real capabilities and limitations while maintaining realistic expectations about its development path.